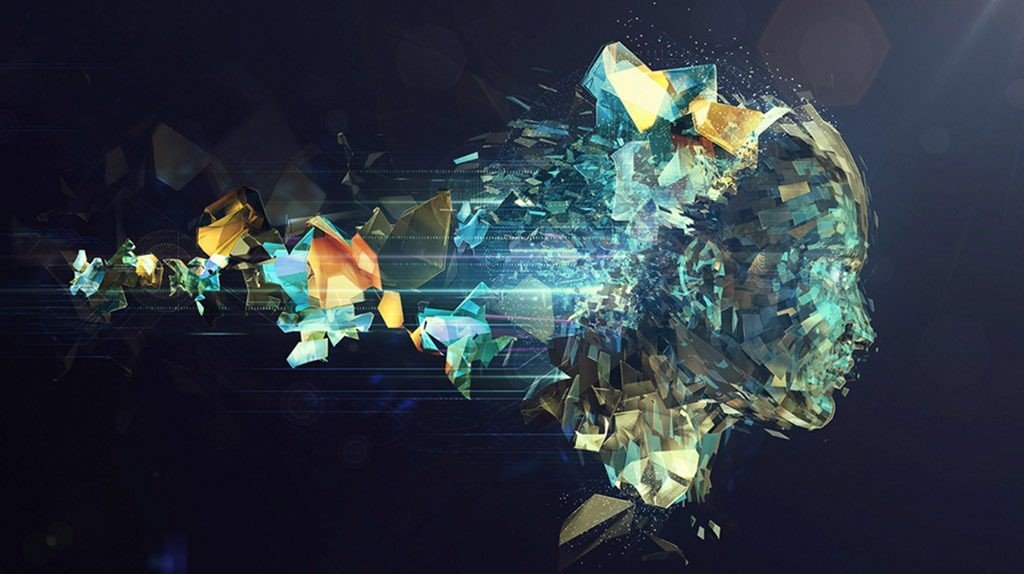
Welcome to the best source of information and research related to Ensemble Active Management (“EAM”)
EAM is a breakthrough approach to active management that emerges when time-tested Machine Learning tools are applied to proven investment principles. EAM is NOT about building a smarter portfolio manager through AI, but approaches investment management from a fresh, more powerful direction.
While EAM is a relatively new category of investing, it is also emerging as one of the fastest growing, with annualized net new flows exceeding $1 billion as of September 2024.
Ensemble Active Management vs ‘Traditional’ Active Management
For more than a half century, the industry’s traditional approach to active management featured:
A single investment portfolio;
Managed by a single manager or team;
With a dedicated investment philosophy and methodology.
EAM rejects this traditional investment design
Instead, EAM portfolios are built on three foundational concepts:
Use of multiple underlying actively managed investment portfolios.
Where the stocks selected are based upon:
Identifying each manager’s highest conviction stock selections (i.e., their best ideas).
Select the stocks with the highest degree of consensus agreement across the underlying managers’ high conviction picks.
EAM portfolios are built using consensus agreement across managers’ high conviction stock selections, and have been proven to generate excess return (see Research Validating EAM, below). The excess return is referred to as Ensemble Alpha.
Why traditional active management hits a glass ceiling
There are countless articles that detail the performance shortfalls of active management. But what is often missing is that there is a mathematical explanation as to why traditional active management consistently falls short:
Mutual funds are, in essence, predictive engines (i.e., managers pick stocks that they ‘predict’ to outperform);
Single engine predictive models fall victim to the Bias-Variance Conflict.
Bias-Variance Conflict is not a theory, it is proven math.
It impacts all single engine predictive models by creating a glass ceiling on predictive accuracy (or for investment portfolios, a ceiling on relative performance). Fortunately, there is a solution – a subset of Machine Learning known as Ensemble Methods (see Ensemble Methods Explained).
The power of
Ensemble Methods
Ensemble Methods improve accuracy in forecasting by mathematically integrating multiple predictive models based on areas of consensus agreement.
Ensemble Methods is also the only known means of offsetting the Bias-Variance Conflict. See Bias-Variance Conflict Explained.
The most well-known application of Ensemble Methods is hurricane landfall predictions, but a simple search on Google Scholar for “Ensemble Methods” and “Ensemble Learning” will show more than 250,000 papers detailing how Ensemble Methods solves complex problems, from facial recognition to cancer diagnosis.
Ensemble Methods have been described as “the most influential development in Data Mining and Machine Learning in the past decade.”
Put simply, EAM is built on the power of Ensemble Methods, and is how mathematicians and data scientists would build investment portfolios.
EAM builds on proven
investment principles
The second core concept underpinning EAM is to identify manager’s highest conviction stock selections. This critical step is supported by substantial research proving that the most reliable source of alpha from active managers is their high conviction stock picks. In almost all studies, high conviction stock selections are defined as the largest overweight positions vs the stock’s weight in the targeted benchmark.
The research supporting this fact is substantial
(see High Conviction Stock Selections).
Two of the more powerful studies include:
2021 paper “Best Ideas” [SSRN-1364827].
M Anton, R. Cohen, C. Polk.
Analysis covered all SEC-registered US domestic equity funds from January 1983 to December 2018, based on analysis of published quarterly holdings.
Key excerpt from paper:
“The stocks in which active mutual fund [ ] managers display the most conviction towards ex-ante, their ‘Best ideas,’ outperform the market, as well as the other stocks in those managers’ portfolios, by approximately 2.8 to 4.5 percent per year”
2019 CFA Institute paper “The Active Manager Paradox: High Conviction Overweight Positions.” A Panchekha.
Analyzed 400,000+ individual rolling one-year performance periods from January 2014 through July 2019.
Reflected real-time holdings data from 114 US equity funds, 57 fund families, with $2 trillion in fund assets.
Key excerpt from paper:
“High-Conviction Overweights, composed of the managers’ best ideas, is the only category that delivers stock selection alpha.”
Research validating EAM
There have been two published White Papers focused on EAM, the first published by the EAM Research Consortium in 2018, and the second by Turing Technology in 2024. The latter was then independently validated by a team of academics at the Georgia Institute of Technology.
Both White Papers and the Georgia Tech validation paper are available for download at EAM Research.
The 2024 White Paper
This White Paper generated 60,000 randomly constructed EAM portfolios, using 333 funds from 140 fund firms, reflecting $3 trillion in fund assets. The evaluation time period was January 2016 to December 2022, and generated more than one-half billion data points.
The Paper focused on relative performance of the EAM portfolios vs the corresponding benchmark over rolling time periods. (See chart.)
The EAM “success rate” in outperforming the benchmark ranged from 74% to 97%.
The annual excess returns averaged 5.0% +/- 0.4%, or 500 basis points +/- 40 basis points.
The Paper’s risk data focused on the likelihood of underperforming the benchmark (i.e., tail risk) for both EAM portfolios and for the underlying mutual funds used in the study.
Key Findings include:
EAM portfolios had a reduced risk of underperformance for rolling 1-year periods vs that of the funds: 27% for EAM vs 45% for mutual funds.
For rolling 5-year periods, the difference in the frequency of shortfall was even greater: 15% risk of underperformance for EAM vs 42% for mutual funds.
Georgia Tech Validation Paper
A team at Georgia Tech were provided complete transparency to the White Paper’s methodology, inputs, outputs, and even code. They then conducted a multi-month evaluation effort. Their work was published January 2024 (“Methodology, Design, and Data Integrity Validation Study of Turing Technology’s 2024 Ensemble Active Management White Paper.” D. Goldsman lead author).
Key excerpts include:
“The EAM portfolio has an overall expected performance benefit of 400–500 basis points [versus] the corresponding benchmark.”
“EAM performance is comparatively better than traditional active management and standard industry benchmarks.”
Why haven’t we seen EAM portfolios until now?
The first known EAM portfolio was put into live production in late 2018 by Bell Family Partners, utilizing technology provided by Turing Technology Associates, and nearly 100 EAM portfolios are known to be in live production, but it wasn’t until late 2023 that EAM first began finding commercial acceptance.
Momentum in 2024 has accelerated with firms launching EAM portfolios as Separately Managed Accounts, ETFs, offshore structured notes, and Collective Investment Trusts.
Total estimated assets in vehicles meeting the definition of EAM is $1.1 billion as of September 2024, and annualized new flows into EAM vehicles has exceeded $1 billion.
Structural obstacles.
EAM requires multiple underlying portfolios, high conviction stock picks, and determining consensus agreement.
For investments, this means multiple ‘underlying’ funds, and then extracting, on a real-time basis, managers’ largest (overweights vs the benchmark).
There are two structural obstacles that have hindered EAM’s development:
1. A multi-manager platform challenges convention regarding how investments are built and distributed. (And, in many cases, runs counter to firm's compensation and promotion structures for individual investment professionals.)
2. Accessing real-time overweight positions requires real-time holdings data, which is always held as a closely guarded secret.
What has changed?
Innovation requires a spark, after which the insight often becomes intuitive. In recent years, that spark has emerged from several sources:
1. One of the world’s largest investment firms built EAM portfolios using their own internal managers. It is estimated that less than a dozen firms have a large enough fund platform to go this route.
2. Firms can access third party managers’ holdings data through mutual agreement. There is one consulting firm that is believed to have begun this approach, but no known products have yet been launched.
3. A technology firm has built a means of replicating (i.e., cloning) active funds with enough accuracy to build EAM portfolios. This is the source of the largest number of EAM portfolios.
Are EAM portfolios more than multi-managed portfolios?
They are; EAM portfolios are very different from multi-manager portfolios and even concentrated versions of multi-manager portfolios.
Multi-manager portfolios take individual investment portfolios and treat them as ‘sleeves’ within a larger portfolio. The primary benefit of them is the ability to reduce overall risk through process diversification. However, mathematically, the return of the multi-manager portfolio is the weighted average of the individual ‘sleeves,’ and thus does not generate incremental alpha vs. the average of the underlying managers.
The missing ingredient in all multi-manager portfolios is the application of Ensemble Methods and the use of consensus agreement to extract the best-of-the-best holdings. That is why EAM has been shown to generate 3% to 5% of annual excess return versus traditional benchmarks (see Research Validating EAM, above.)
Footnotes:
1 Turing Technology Associates
2 Documents referenced:
“AI-Powered Active Management (EAM) is Poised to Dominate Passive – Here’s How and Why.” February 2024. Published by the CFA Institute, Lead authors Fishman, Nestor.
“EAM – AI’s Transformation of Active Management.” White Paper published January 2024. Published by Turing Technology Associates, Lead authors Fishman, Nestor.
“Methodology, Design, and Data Integrity Validation Study of Turing Technology’s 2024 Ensemble Active Management White Paper.” January 2024. Lead author D Goldsman, Coca-Cola Foundation Professor and Director of Master’s Programs for the H. Milton Stewart School of Industrial and Systems Engineering, Georgia Institute of Technology.
“Ensemble Active Management – The Next Evolution in Investment Management.” White Paper published September 2018. Published by Turing Technology Associates, lead author A Panchekha.
3 Documents referenced:
“Why Active Fund Managers Often Underperform the S&P 500: The Impact of Size and Skewness.” D Ikenberry, R Shockley, K Womack, Portfolio Management, 1998
“Underperformance of Actively Managed Portfolios: Some Behavioral Insights.” E Otuteye, M Siddiquee - Journal of Behavioral Finance, 2020
“The Dimensions of Active Management.” MB Waring, LB Siegel - Journal of portfolio management, 2003
“Active vs. Passive management: New Evidence From Exchange Traded Funds.” GG Rompotis ,2009
“Rating the Performance of Active Equity Fund Managers.” P Hodgens, P Dolan, G Wells - JASSA, 2001
“Global Equity Fund Performance, Portfolio Concentration, and the Fundamental Law of Active Management.” J Huij, J Derwall - Journal of Banking & Finance, 2011
“Does Active Management Pay? New International Evidence.” A Dyck, KV Lins, L Pomorski - Review of Asset Pricing Studies, 2013
“The bad arithmetic of active management.” BJ Jacobsen - Journal of Portfolio Management, 2017
“Performance Drawdowns in Asset Management: Extending Drawdown Analysis to Active Returns.” D Bradford, D Siliski - Journal of Performance Measurement, 2016
“Fund Manager Conviction and Investment Performance.” L Jin, R Taffler, A Eshraghi, OK Tosun - International Review of Financial, 2020
“Active Share and Mutual Fund Performance.” A Petajisto - Financial Analysts Journal, 2013
“Performance Implications of Active Management of Institutional Mutual Funds.” R Bird, P Pellizzari, D Yeung - Accounting & Finance, 2015
4 “Ensemble Methods in Data Mining.” 2010. G. Seni and J. Elder, Morgan and Claypool
5 Relative performance Success Rate is defined as the percent of times that a strategy outperforms its benchmark over rolling one-year periods. A Success Rate of 100% is the best score, a Success Rate of 0% is the worst.
6 Turing Technology Associates
7 Turing Technology Associates
8 Turing Technology Associates
9 Turing Technology Associates
10 Turing Technology Associates
11 Documents referenced:
“Fund of Funds Investing – A Roadmap to Portfolio Diversification.” 2010. D Strachman and R Bookbinder, John Wiley & Sons
“Risk Diversification in Multi-Manager Products.” 2007. S Rich, MoneyManagement
“Pursuing returns while managing risk: the potential benefits of multi-manager portfolios.” 2022. S Keshishighli, Mercer